Newsletter #3: Products, Security, ML Azure Pipelines & More
Understand best practices for building real world machine learning systems
Welcome to another edition of the Hub of Machine Learning's Newsletter. ✉️ If you missed the last one, you definitely should catch up here.
This month I came across some interesting posts 🎉 on products, security, recommender systems, etc. that I thought were worth sharing with you.
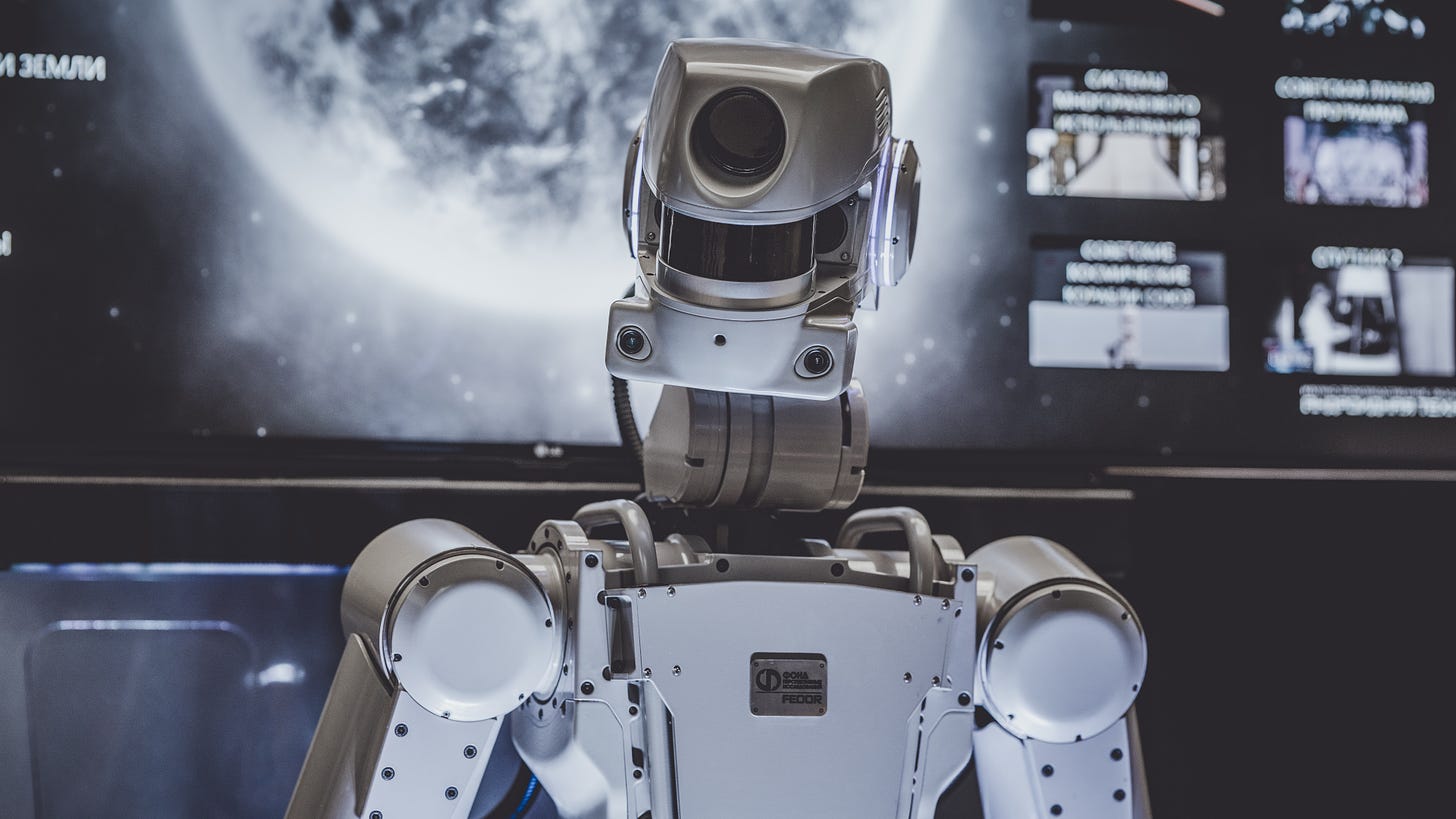
Photo Credit: Unsplashed
Building Machine Learning Products
Product requirements can be given vaguely at times, without asking some thoughtful questions to understand what problems a machine learning product should solve and how; one might be setting himself up for failure. Read Jeremy Jordan's post on building a machine learning product.
How to Apply Machine Learning to Solve Business Problems
One of the key benefits of applying machine learning is gaining a competitive edge in the market. How can you apply machine learning to solve business problems? Learn more.
Machine Learning in Production
This is a five series post by Luigi Patruno on what it means to deploy an ML model, what factors to consider when implementing models, what software development tactics to use, and the tools and frameworks to utilize.
Stealing Modern NLP Systems with Gibberish
Model fine-tuning is a popular approach in natural language processing. I came across a post by Kalpesh Krishna about security implications for NLP models trained using BERT.
How I built an Intelligent Bot
Twitter users tweet 500 million tweets per day. The volume of information going through Twitter per day makes it one of the best platforms to get information on any subject of interest. This post walks you through how I built a twitter bot powered by machine learning.
Estimating a Classifier Performance Just by Looking at the Data Distributions
The quality of your data goes a long way in determining the performance of your model. Understanding how to explore your data before training can save you a lot of valuable time. This post shows you how to estimate a classifier's performance from the data distribution.
Building a Repeatable ML Workflows with Machine Learning Azure Pipelines
Machine learning involves complex workflows of data preparation, training, hyper-parameters tuning, evaluation, and tuning. Janakiram MSV wrote an interesting post on building a repeatable machine learning workflows with Azure pipelines.
Exploring Transfer Learning with T5: The Text-To-Text Transfer Transformer
Explore the landscape of transfer learning techniques for NLP by using a unified framework that converts every language problem into a text-to-text format.
Build a Recommendation Engine with Collaborative Filtering
Collaborative filtering is one of the most common techniques used when it comes to building intelligent recommender systems that can learn to give better recommendations as more information about users is collected. This post shows how to build a collaborative recommender system.
PyTorch: A Step-by-Step Tutorial
PyTorch is one of the fastest-growing deep learning frameworks. People choose PyTorch because of its simple, similar syntax to Python. This post by Daniel Godoy is a place to start if you're just getting started with PyTorch.
Google Open Sourced a Framework for Quantum Machine Learning
On March 9th, Google, in collaboration with the University of Waterloo, X, and Volkswagen, open-sourced TensorFlow Quantum (TFQ), a library for the rapid prototyping of quantum ML models. The framework offers high-level abstractions for the design and training of both discriminative and generative quantum models under TensorFlow and supports high-performance quantum circuit simulators.
Thanks for reading! If you like this newsletter and want to support it, click on the image below to buy me a $2 coffee.
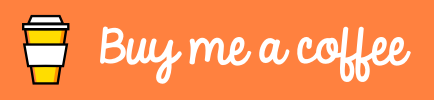
Cheers,
Samuel